Machine learning is a type of artificial intelligence that improves its performance based on the data available to it. Lately, the use of ML has been growing in medicine, where it’s been introduced to solve many time-consuming and complicated tasks. In this article, we will describe how healthcare and machine learning fit together, including the tech’s applications, use cases, challenges, and benefits. We will also show you how to implement machine learning into existing solutions and create robust machine learning apps to benefit from this revolutionizing technology.
Impact of Machine Learning in Healthcare
First, let’s take a look at the application of machine learning in healthcare.
Classification
Classification involves separating the data into groups for further use. The most obvious use for it is in medical imaging; for example, to mark healthy versus cancerous cells.
Recommendations
Machine learning-based systems can make suggestions to help users. In the medical sector, this can be used for supporting clinical decision-making. Once a doctor fills out a patient’s information in the electronic health record, the system will show similar cases, treatment protocols, and results.
Clustering
Clustering is similar to classification. It also groups similar information but doesn’t assign any specific labels. This approach is often used for various kinds of medical research, from sifting through clinical data to finding new medicines.
Prediction
Based on current and historical data, artificial intelligence can make reliable predictions. This covers more than just medical information — predictions can be made about the patient numbers, revenue, and other business metrics.
Anomaly detection
As the name suggests, this approach means finding things that are out of the ordinary. On the one hand, it helps diagnose diseases at early stages, only judging by the images and vitals. On the other, it can pinpoint irregular or unusual customer behavior that may indicate insurance fraud.
Automation
Medical professionals can spend two or more hours per day working with EHRs. More than half of them find this task annoying. Machine learning can help automate many routine and repetitive activities, thus leaving clinicians more time to spend with their patients and decreasing doctor burnout rates.
Looking to acquire the gains listed below? Machine learning can deliver them! Send us a message and schedule a free consultation.
Benefits of Machine Learning in Healthcare and Med Tech
Machine learning applied to healthcare brings the following benefits.
Accurate Data
ML-powered software can aggregate the information that your employees enter, clean it up, and check for possible mistakes. This helps maintain your medical records, keeping them up to expected standards, as well as set the groundwork for additional benefits based on the analysis of this data.
Outbreak prediction
By analyzing previous epidemics, new patient symptoms, and unstructured information (e.g. news articles and social media posts), an ML-powered system can warn you about an upcoming outbreak before it happens. You can prepare accordingly — modify staff schedules, procure extra supplies, etc.
Precision medicine
This approach, also known as “stratified” or “personalized” medicine, allows doctors to tailor treatment plans to a specific person. Adaptations can include drug dosage and intake schedule, diet, procedures, exercise regimen, and more.
Thanks to machine learning, this methodology can be accelerated. By gathering as much relevant data (genetic makeup, medical history, daily habits, etc.) as possible and analyzing it, hospitals can assist their doctors in treating each patient based on their own situation, and not solely on the averages around populations or demographics.
Accurate diagnostics
Machines are already on par with human doctors (albeit with a few caveats) when it comes to diagnosing certain illnesses. Existing machine learning algorithms can detect disease biomarkers in blood, identify skin cancer, diagnose colorectal cancer from images — the list continues to grow.
It is a well-developed and powerful application of machine learning for healthcare projects. So hospitals looking for healthcare software development services often include it in their requirements.
Easier drug discovery & development
Finding new medicines, testing them, and getting them to market is a lengthy and expensive process. Machine learning can assist in all its stages: finding materials, synthesis, data mining, target validation, etc. Overall, this massively speeds up drug development in silico and allows pharmaceutical companies to get better ROI on their discoveries.
Improved clinical trials
Machine learning can make considerable difference when it comes to testing medicines. ML can find the most fitting patients to participate in a trial, improve adherence and retention, and thoroughly analyze the results. More advanced algorithms can even find data that is not explicitly mentioned by the patients by tracking clues in their medical records.
Robot-assisted surgery
The machines can’t yet perform operations on their own. But there are plenty of examples where they assist humans in this task. They can suture tiny blood vessels, act as scrub nurses, harvest hair follicles for transplantation, and more.
Robots can also act as more precise intermediaries for surgeries where extreme accuracy is required, such as during eye surgeries. In this case, a specially trained human doctor controls a robot that performs the tasks without even the slightest tremor occurring.
Faster data entry
We have already mentioned the problem of physicians spending too much time in an EHR/EMR. A large part of it is due to entering data from the patient visit and analyzing documents. However, with Natural Language Processing and Optical Character Recognition, this can be done much more quickly. The computer will interpret clinical notes or recognize the words spoken during the visit and extract out the relevant information.
As a result, medical professionals will do medical work, while the machines handle the clerical tasks.
Improved administrative processes
Machine learning applications in healthcare go beyond just health-related issues. They are beneficial to the business side of things as well. ML systems can predict trends, analyze business data to assist in informed decisions, automatically collate reports for shareholders and owners, and automate scheduling.
In this regard, medical businesses aren’t that different from their counterparts in other verticals. So the common enterprise applications for machine learning work for them just as well
Want a comprehensive ML solution that benefits both clinical and administrative sides of your practice? Schedule a consultation with our experts, and let’s discuss it.
Opportunities of Machine Learning in the Medical Field
Using AI and machine learning in the medical field opens an entire host of opportunities to all tech-savvy practices and startups.
ML and AI empower both diagnosis and recovery
In the field of medical care, machine learning can help determine disease faster, and speed up recovery in patients.
Oncology is one of the specialties where computer-assisted diagnostics is more popular. There are public health datasets for machine learning to train breast cancer-detecting software, DeepGene, an advanced deep neural network-based classifier, which accurately diagnoses somatic mutations, and other artificial neural networks (ANNs) that predict optimal treatment outcomes.
That is not to say that other areas don’t benefit from machine learning. Diagnostics of Parkinson’s disease, for example, or various skin ailments also turn to AI and ML.
When it comes to recovery, this technology’s main application is predicting the results based on the historical data about the population in general, as well as the specific patient. This has already been used in cardiology, arthrology, oncology, and other fields.
In addition, it can be used to improve adherence. Based on the medical history and other parameters, ML algorithms can determine which patients are more likely to skip taking their drugs and have the physicians pay more attention to them. Medical science shows that such approaches have a proven positive influence on patient outcomes.
All-in-all, this application of ML for health is well-researched and effective.
Surgeries performed by robots
Surgical robots are a major trend in precision medicine. They allow for making smaller incisions, thus shortening the recovery time and drastically decreasing blood loss. In most cases, a robot is an instrument controlled by a surgeon who specializes in the surgery at hand.
However, autonomous surgical robots — ones that can operate without human input — have already shown promise during in vivo and ex vivo tests. STAR (smart tissue autonomous robot) outperformed experienced human surgeons in procedures on porcine intestines. Other AI-controlled robots showed promise in vascular and ocular microsurgery, as well as performing supporting tasks (e.g. fetching the right instruments).
There are also ambitious projects to help doctors and robots learn from each other. Humans can show robots how to perform surgeries safely and effectively, while receiving visual cues and warnings based on tool trajectories, for example.
Robotic surgery is one of the more futuristic areas of medical machine learning projects, but it is also one of the most promising.
Medicine research and manufacturing
One of the most common medical applications of machine learning is drug discovery, research, and manufacturing. Major pharmaceutical companies like Pfizer, Novartis, and Merck use it to make finding, developing, and testing new medicines faster and easier.
While this data-driven approach is relatively new, 15 small-molecule drugs developed with ML are already in clinical trials, and 150 more are on the way. What’s more, this trend shows a massive 40% year-on-year growth.
Overall, using ML for drug discovery and research has many benefits:
- Predicting the efficiency of a medicine. Based on the chemical composition of a drug, the system can accurately guess whether it would be effective and how large of a dose will be needed.
- Predicting the possible side effects. This helps the company to cut their losses and work on a more promising product
- Reporting and compliance management. Automatically generating reports for scientific and administrative personnel, as well as creating the documents that are required by law.
- Rapid iterations. Testing many potential candidates in silico and selecting the ones with the most potential.
Investment in ML medical startups focused on drug discovery and development has also been growing. It reached $2.1 billion in the first half of 2021 alone. Some of the largest investment rounds were ones for Cellarity ($123 million), Dyno Therapeutics ($100 million), and Enveda Biosciences ($51 million).
Top 5 Machine Learning Applications in Healthcare
In this section, we will look at some typical machine learning use cases in healthcare.
Clinical decision support systems
Clinical decision support systems (CDSSs) make the most of healthcare data for machine learning. They are software that analyze the information in EHRs and help doctors provide more effective patient care. Studies have produced plenty of evidence proving their effectiveness in cardiovascular, pulmonary, and other fields. Using CDSSs is considered a best practice by the Centers for Disease Control and Prevention (CDC).
The aids such systems can provide are many: “computerized alerts and reminders to healthcare providers and patients; clinical guidelines; condition-specific order sets; focused patient data reports and summaries; documentation templates; diagnostic support, and contextually relevant reference information, among other tools,” as stated by the Office of the National Coordinator for Health Information Technology (ONC).
The clinical results that CDSSs bring are impressive. They include decreasing sepsis mortality by over 50%, 52% reduction in readmissions, and 42% reduction in ED visits.
Besides improving patient outcomes, a CDSS positively affects the clinic’s cash flow. As a case study of South Omaha Medical Associates shows, since implementing such a system, the practice saw a 25% rise in patient visits due to better patient monitoring (including using information from personal blood pressure cuffs) and diagnostics. And a veteran’s affairs site in Indiana saved $150K per year by optimizing the use of their lab facilities.
Smart recordkeeping
Smart recordkeeping is a concept that combines data management, compliance, and performance management. It is inherently connected with other software that a practice uses: EHR/EMR, PMR, ERPs, scheduling systems, and others, and relies on the data that they provide. In addition, a smart recordkeeping system can share the information with third parties (e.g. insurance companies or government agencies).
Software like this streamlines everything that has to do with access to patient and business information, as well as acts as a risk-mitigation tool. For example, it eliminates duplicates of patient data, prevents treatment and billing errors, maintains the veracity of the information, creates backups, etc.
As an additional benefit, smart recordkeeping systems can collate reports and maintain an audit trail — an important part of being legally compliant.
Medical imaging
Machine learning healthcare applications include solutions for medical imaging. They are used to assist in analyzing X-rays, СT scans, and other 2D and 3D images.
Machine learning helps analyze images. Source
This application saves a lot of time for radiologists, pathologists, and other relevant specialists. Software needs only a few seconds to do the work that would take a human many minutes or even hours. Machine learning algorithms screen for cancer, detect atrial fibrillations, and epileptic seizures.
There are open-source examples as well. Microsoft’s InnerEye provides the groundwork for researchers and businesses to create their own medical diagnostic tools based on images.
And there are consumer-facing implementations. Google’s DermAssist is one — the app helps people determine the skin problem that they have (although they still should visit a doctor for a solid diagnosis). Companies that don’t have such deep technological expertise can retain healthcare app development services from a trusted provider.
Personalized treatment
Tailoring a treatment plan to a specific person has long been thought of as a holy grail in medicine. Machine learning algorithms used in healthcare has brought this closer to reality. As this niche is incredibly complex, it is still in its infancy, but certain practices are already using it.
Such an approach provides a host of benefits:
- Locating patients that are at a higher risk and prioritizing them
- Identifying underserved patients
- Maintaining patient connections
- Spending medical professionals’ time better
- Decreasing the risks for human error
With all these benefits, personalized medicine is the medicine of the future.
Clinical trial and research
Testing new drugs and devices is arduous. A company has to find fitting participants, retain them, invest millions, establish a high success rate (failure is not an option), and that’s not all the potential troubles. About 90% of clinical trials fail.
With machine learning algorithms in healthcare, the situation can be significantly improved.
First, the algorithms can assist with trial design. By analyzing available information (previous trials, research articles, etc.) they can present actionable patterns to base the trial on.
Second, they can help the clinical team select the most fitting patients to participate and help them stay on for as long as possible. The best participants will be chosen based on their medical data pulled from EHRs, wearables, and other relevant sources. Then the system will track their activities and offer support to prevent dropouts and ensure safety.
Finally, ML can assist in analyzing the acquired data. The trial results can come from separate sources and in different formats, but with the right algorithms, even the unstructured data can be filtered for important information. Sifting through mountains of data is the task this technology was created for.
If you need a powerful CDSS, a smart recordkeeping system to keep your documentation in order, or an application to assist with clinical trials, EPAM Startups & SMBs is your best bet. Schedule a free consultation now.
Ethics of Using Machine Learning in Healthcare
Using machine learning in hospitals requires a conscious approach to, and mindfulness of, certain ethical issues.
Privacy and data security
This is a common issue across the entire Healthcare IT domain.
Protected health information requires the utmost protection. On the one hand, it commands the highest price on the black market because it is easier to connect with a specific person than most other data types. On the other, it is extremely sensitive and can lead to serious harm to one’s reputation or health. This is true, for example, in case of drug/alcohol addiction, AIDS, or abortion.
To address this, the company has to use both technical and organizational measures. The former include pseudonymization, encryption, firewalls, and antivirus software. The latter include access to the information on a need-to-know basis (role-based access) and monitoring potential leak points.
Fairness
You can’t make a good knife out of bad steel. Neigher can you make an effective machine learning system without a good, balanced dataset. The term refers to the collection of data that is used to effectively train algorithms. This means it should encompass the broadest scope of potential cases in the right proportion.
The datasets should reflect the true epidemiological situation across all demographics and contain enough members of each stratum to be effective. Otherwise, it will make wrong decisions.
Transparency
Machine learning is an advanced technology that is often hard to understand for a non-technical person. Moreover, the specific algorithms used in each piece of software are different. As a result, doctors and patients inherently distrust the “black box” that makes important clinical decisions.
Patients and medical professionals need to understand at least the gist of how the ML system works to ensure informed consent and effective use of the software.
Challenges of Machine Learning in Healthcare Projects
Implementing a complex technology, like merging machine learning and healthcare, is inherently hard. Anyone who wants to embark on this journey needs to be aware of potential hurdles in the way.
Lack of quality data
Garbage in / garbage out is a computer science maxim that is most fitting for ML. Machine learning techniques require a lot of quality information. Without it, your system will not be able to make the right decisions. But getting this data is a major challenge, especially when it comes to medicine.
A lot of problems can arise:
- Typos. Misspelled words, missing dividers in numbers, and other similar instances can create errors that affect the entire dataset.
- Missing information. Sometimes, certain data simply doesn’t enter the system, e.g. because the provider was suddenly distracted.
- Duplicates. Suppose a patient got a prescription that is covered by insurance. If your ML system pulls data from both e-prescriptions and insurance claims, the information could end up doubled.
- Format misalignment. If you need to gather data across multiple different systems: medical databases, EHRs, wearable devices, etc., it might come in different formats. Your ML software might struggle to parse it.
- False info. “Everybody lies,” said a medical genius from a certain show. This includes people entering data.
- Unbalanced data. Information that doesn’t present all demographics and conditions in a large enough proportion.
- Small size. Compared to other niches (e.g. computer vision), medical datasets are inherently smaller. After all, it is easier to get a million selfies than a million psoriasis patient records. This means a less reliable training process for the algorithms.
These issues are common across all niches that use big data. So if you want to hire a contractor to develop your ML project, make sure they have experience providing big data development services.
Other ways to solve data quality issues include manually curating the information for the dataset and using proven public datasets (if there are relevant ones).
Regulations (HIPAA, MACRA, GDPR, etc.)
Given this industry’s importance, governments and multinational organizations have implemented a lot of laws that directly or indirectly affect how machine learning is used in healthcare. They include HIPAA, GDPR, MACRA, and many others. Complying with them presents an additional challenge to hospitals and medical startups.
Data protection measures taken to comply with the regulations can slow down the software (for example, by making it decrypt encrypted data).
High cost of error
In medical ML, any mistakes can be life-threatening. For example, suppose a woman came in for breast cancer screening. The algorithm analyzes her ultrasound images and finds no problem, so she leaves, content. Meanwhile, it was a false negative and there is an early-stage tumor. Starting treatment earlier could’ve given the patient a higher chance of recovery, not to mention saving her a lot of money on treatment.
This means that the accuracy of ML algorithms has to be much more rigorous than in other domains.
Predicting ROI
Machine learning is a powerful technology, but its effectiveness varies from task to task. In addition, this effectiveness changes with time and is different from project to project. This means predicting the returns becomes next to impossible, and implementation risks go up.
Shiny object syndrome
This sounds medical, but it’s actually a problem for all new technologies and across all industries. ML is trendy and powerful, and many respectable and successful healthcare businesses use it to gain benefits. Looking at them might cause a fit of FOMO and a rush to implement it at your own practice.
This is wrong. Machine learning is a tool, and just like any tool, it requires the right job. Perhaps it isn’t even right for you, or the implementation costs outweigh potential benefits. Thorough analysis now translates to optimal ROI later.
EPAM's Experience Using Machine Learning in the Medical Field
Specialists working for EPAM have experience in many medicine-related niches, from medical website design and development services to software for managing chronic conditions and massive insurance portals. Blending machine learning and health data is something well within their collective skill sets.
One example is webPOISONCONTROL, an app for triage and managing poisoning. A user can get reliable information on symptoms and threat level depending on their age, weight and other characteristics — all enabled by an ML-powered recommendation engine. Then the app can either suggest they immediately go to the ER, call an ambulance or stay home. In addition, it can quickly recognize pills based on their looks or barcodes.
Another one is RaySafe, a patient-focused app that helps manage exposure levels from X-rays. It provides a simple way for doctors and nurses to collate information on how much radiation a certain person absorbs, getting this information straight from the X-ray machine. Doing so reduces unnecessary scans, as well as automatically notifying doctors in cases of excessive exposure.
Conclusion
Healthcare and machine learning are a natural combination. Medical practices that employ ML obtain many advantages: better patient outcomes, streamlined administrative processes, decreased physician burnout, and enhanced accuracy of the information concerning their patients and epidemiological trends. Pharmaceutical companies get faster and more efficient R&D, as well as accelerated trial timelines.
There are certain challenges, like gathering reliable data or compliance with regulations. But overall, the benefits far outweigh the risks.
If you see machine learning as a technology that could help you, call us or send a message — the first consultation is free.
FAQ
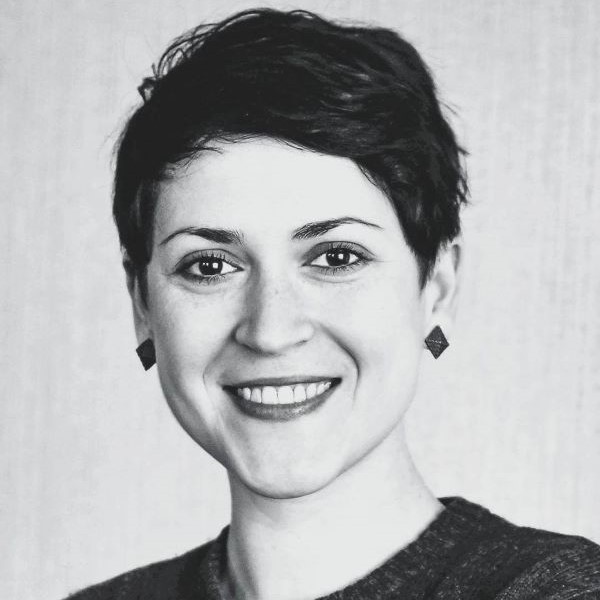
Expert digital communicator and editor providing insights and research-based guides for technology buyers globally.
Expert digital communicator and editor providing insights and research-based guides for technology buyers globally.
Explore our Editorial Policy to learn more about our standards for content creation.
read more