What is Computer Vision in Healthcare?
As surprising as it may sound, computer vision goes back as far as the 1960s, when computers started appearing at universities and scientific labs in large numbers. Back then, the growing infatuation with cybernetics and robotics paved the way for the emergence of several important disciplines, including artificial intelligence and computer vision.
However, it wasn’t before the turn of the millennium that computer vision concepts started becoming a reality and were implemented in specific products and services. From mobile devices and access control systems with facial recognition features, to powerful AI-enabled CCTV surveillance systems installed in nearly all major cities, to Tesla’s fleet of cars with autopilot capabilities, computer vision is now a part of our reality.
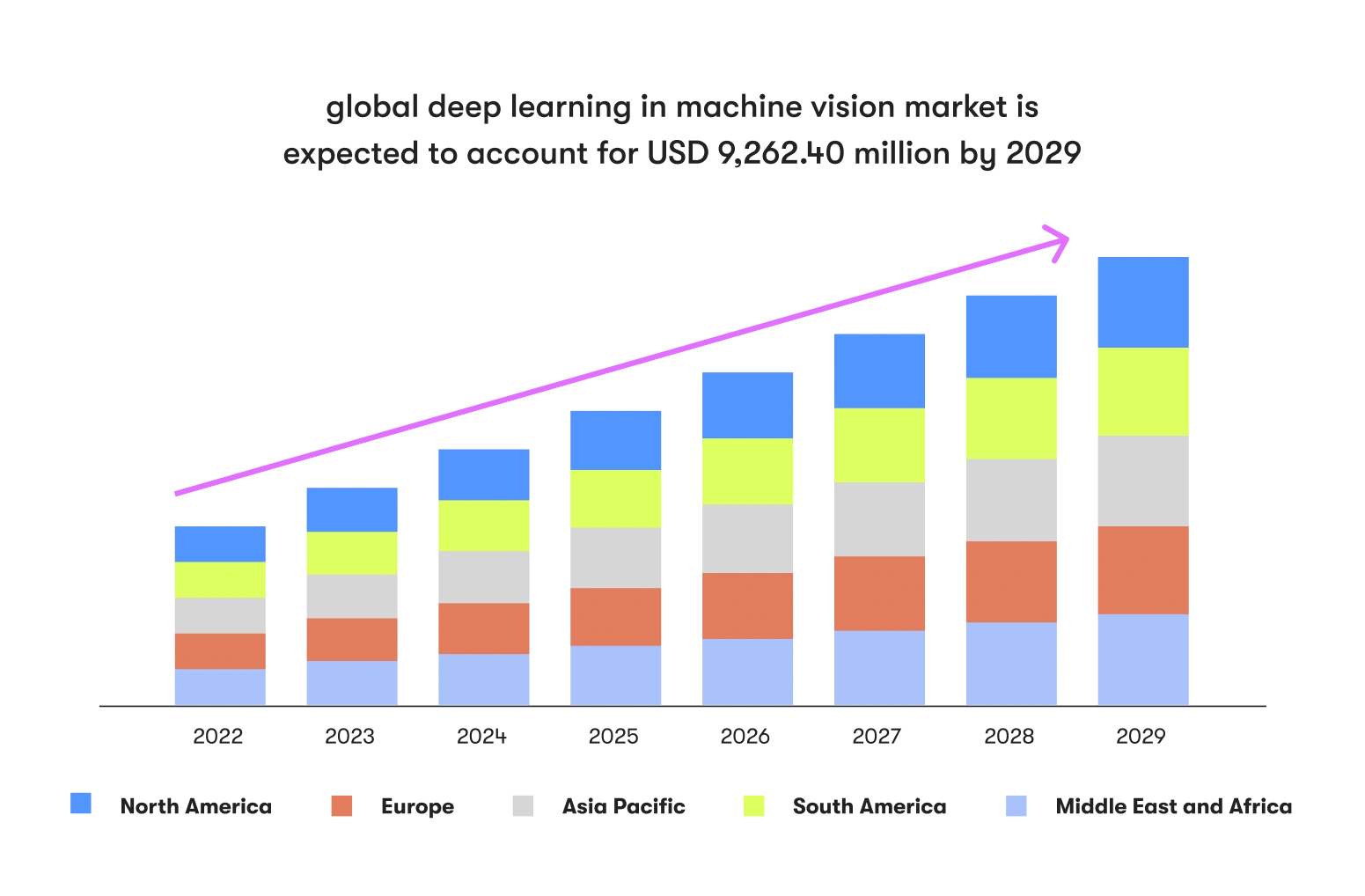
Healthcare was one of the first industries to recognize the immense potential of computer vision and the convolutional neural networks (CNNs) powering the technology. Application of computer vision in the medical field is based on leveraging the capabilities of artificial intelligence and deep learning in a variety of contexts. Let’s name just a few of them:
- DICOM image analysis
- Detection of anomalies in MRI, CAT and X-ray scans
- Diagnostic assistance
- Surgical assistance and prevention of inadvertent retention of surgical instruments
- Calculation of blood cells
- Retina scans and early detection of structural changes
- Identification and analysis of new or recurring skin anomalies
- Remote monitoring and patient care
- Post-traumatic rehabilitation
Now that we can see the scope of computer vision use cases in healthcare is significant, let’s take a deeper dive into the nuts and bolts of the technology and examine more closely how the tech is applied in particular areas.
HAVE A PROJECT CONCEPT IN MIND BUT UNSURE ABOUT ITS FEASIBILITY?
Let our engineering team leads and subject matter experts dispel your doubts. With EPAM Startups & SMBs, you’ll have a crystal-clear picture of your options and expected outcomes long before a line of code is written!
How does computer vision work?
Computer vision is a subfield of the broader, overarching term, “artificial intelligence,” and deals with the problem of analyzing still images or video streams with the purpose of understanding their content in order to make conclusions and perform certain actions.
Computer vision relies on highly complex mathematical algorithms (neural networks) that are trained using datasets — collections of images related to particular subject matter. The longer a neural network is trained and the larger the dataset is, the higher the resulting accuracy becomes.
Computer vision has evolved dramatically over the course of just a decade, and has effectively improved its average accuracy from around 50% previously to 99% at present. This can be attributed to the sophistication and optimization of deep learning algorithms and the rapid increase of the volume of digital images on the web that can be used for training neural networks.
There have been major improvements on the performance side as well. Today’s machine vision systems are powered by ultra-fast CPUs with optimized instruction sets, GPUs with hundreds of concurrent pipelines, and even specialized VPUs (Vision Processing Units) capable of accelerating the execution of AI algorithms on the hardware level.
Future of computer vision
Several factors will define the future evolution of computer vision technologies:
- The ever-increasing processing power of mainstream and specialized chipsets will make real-time image recognition and object detection much faster.
- Further development of deep learning (DL) algorithms and the growth of the size and quality of datasets will increasingly boost the precision of computer vision, enabling a much higher level of detail.
- More and more CV-enabled applications will be moving to the edge — that is, working autonomously on terminal devices and providing instant reaction to analysis results without having to wait for data to be processed in the cloud.
So far, we’ve used multiple terms like “artificial intelligence,” “machine learning,” “computer vision,” and “deep learning.” They are closely related, but what makes deep learning “deep”?
Advantages of deep learning in computer vision
In the early days of computer vision, every process was tedious, time-consuming, and required tons of manual labor for image classification, data preparation, data point management and so forth. All of these things made real-time image analysis impossible, while the results were far from consistently accurate.
With time, the emergence of machine learning algorithms, such as linear and logistic regression, decision trees and support vector machines (SVM), enabled software engineers to automate huge chunks of manual operations by packing them into so-called “features” — compact applications capable of tracking down patterns in images much faster and with incomparably greater effectiveness.
However, this wasn’t the end of the story. When deep learning arrived, it changed the entire AI and CV game forever. With convolutional neural networks, engineers received a sophisticated, self-improving mechanism with the ultimate level of automation capabilities, one that only required some fine-tuning and careful preparation to successfully handle large datasets to work on its own.
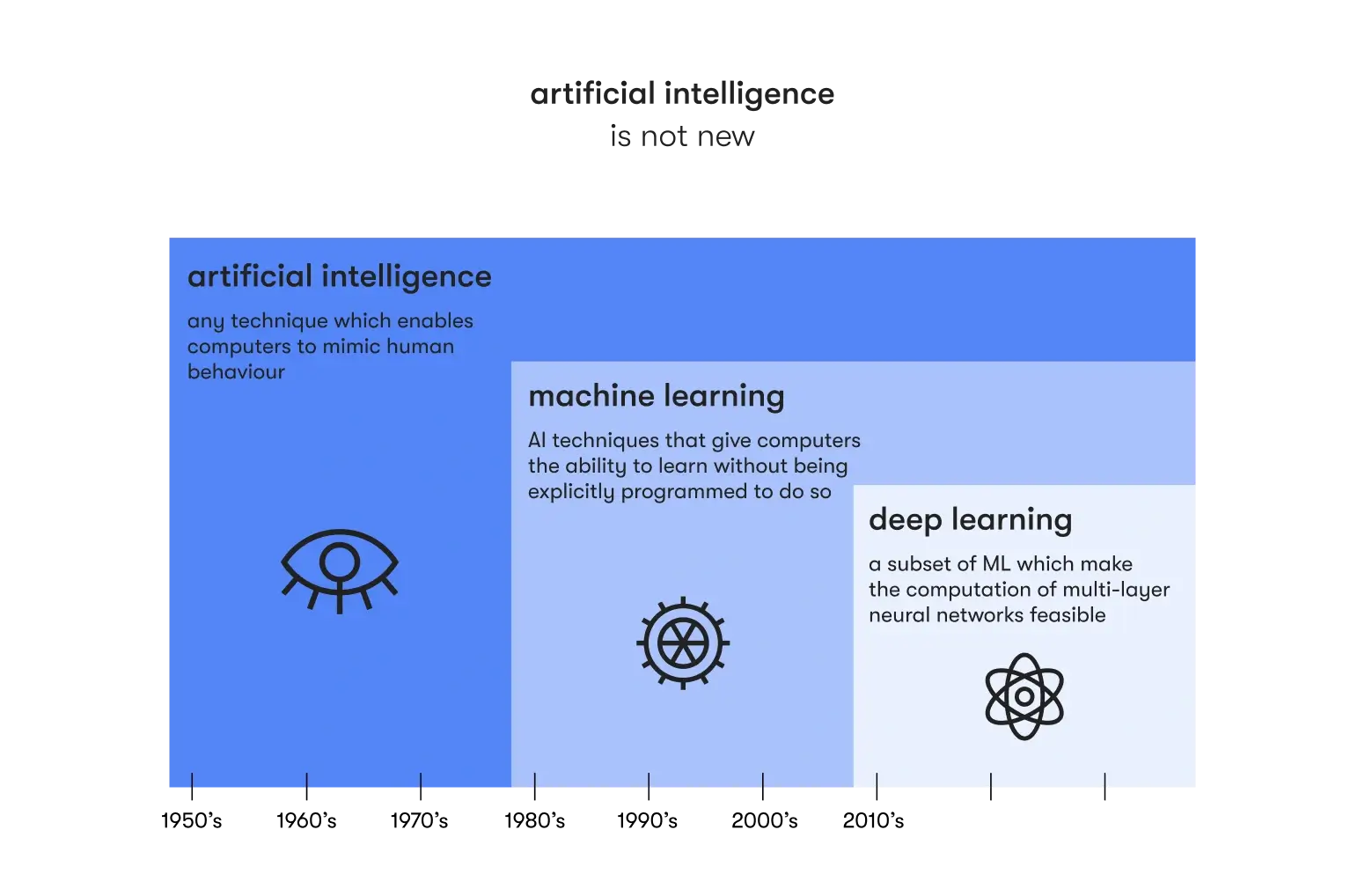
Modern computer vision systems rely on traditional ML and advanced DL algorithms to solve the most complex image analysis and object recognition problems.
Who are the key users of computer vision technology?
The quick answer is any organization developing or operating software/hardware systems interfacing with the physical world with the purpose of detecting objects, people, features, threats or anomalies through the use of image capture devices (cameras) and cognitive algorithms based on the work of a neural network.
A few obvious examples would be:
- Car manufacturers.
- Manufacturers of industrial inspection systems, including drone-based ones.
- Healthcare professionals.
- Manufacturers of security systems.
- Retailers.
- Large venue operators.
- Product developers focused on sports analytics.
- Manufacturers of maps and navigation systems.
- Agriculture companies.
For the purposes of this article, let’s take a closer look at one particular industry teeming with computer vision projects and products of various types.
INTERESTED IN BUILDING A CV-ENABLED SOLUTION BUT DON’T KNOW HOW TO GET STARTED?
EPAM Startups & SMBs is here to help. Just drop us a line, and we’ll get back to you to discuss your business case and propose the optimal approach to fulfilling your goals based on our medical app development services. We have the best engineering talent, exactly what your project deserves.
Benefits of computer vision applications in healthcare
As we mentioned earlier in the article, the healthcare industry is one of the main beneficiaries of the rapid advancement of computer vision technologies. Application of computer vision in healthcare has helped improve a great number of medical disciplines and save thousands of lives, all thanks to improved diagnostics, earlier detection of health issues, and better treatment plans.
Computer vision — what do doctors gain?
Clinicians and patients jointly benefit from the use of computer vision in healthcare applications. On the doctors’ side, CV primarily helps reduce the number of diagnostic errors and false positives by providing a second opinion on diagnostic conclusions and detecting the most minute anomalies and deviations from the norm that can be overlooked by physicians during manual observations.
In addition to more accurate diagnoses, computer vision can be an invaluable asset in surgery wards by helping surgeons and surgical nurses prepare for operations, keep track of surgical instruments before and after, and even assist experienced surgeons in training their younger colleagues.
Improved patient experience and saved lives
For patients, the multiple types of computer vision medical applications translate into faster admissions, access to self-service kiosks, remote health monitoring scenarios and other benefits of medical automation. Most importantly, however, is that computer vision in health-related usage scenarios does help save lives and make treatments less aggressive, traumatic, or expensive.
Computer vision in healthcare
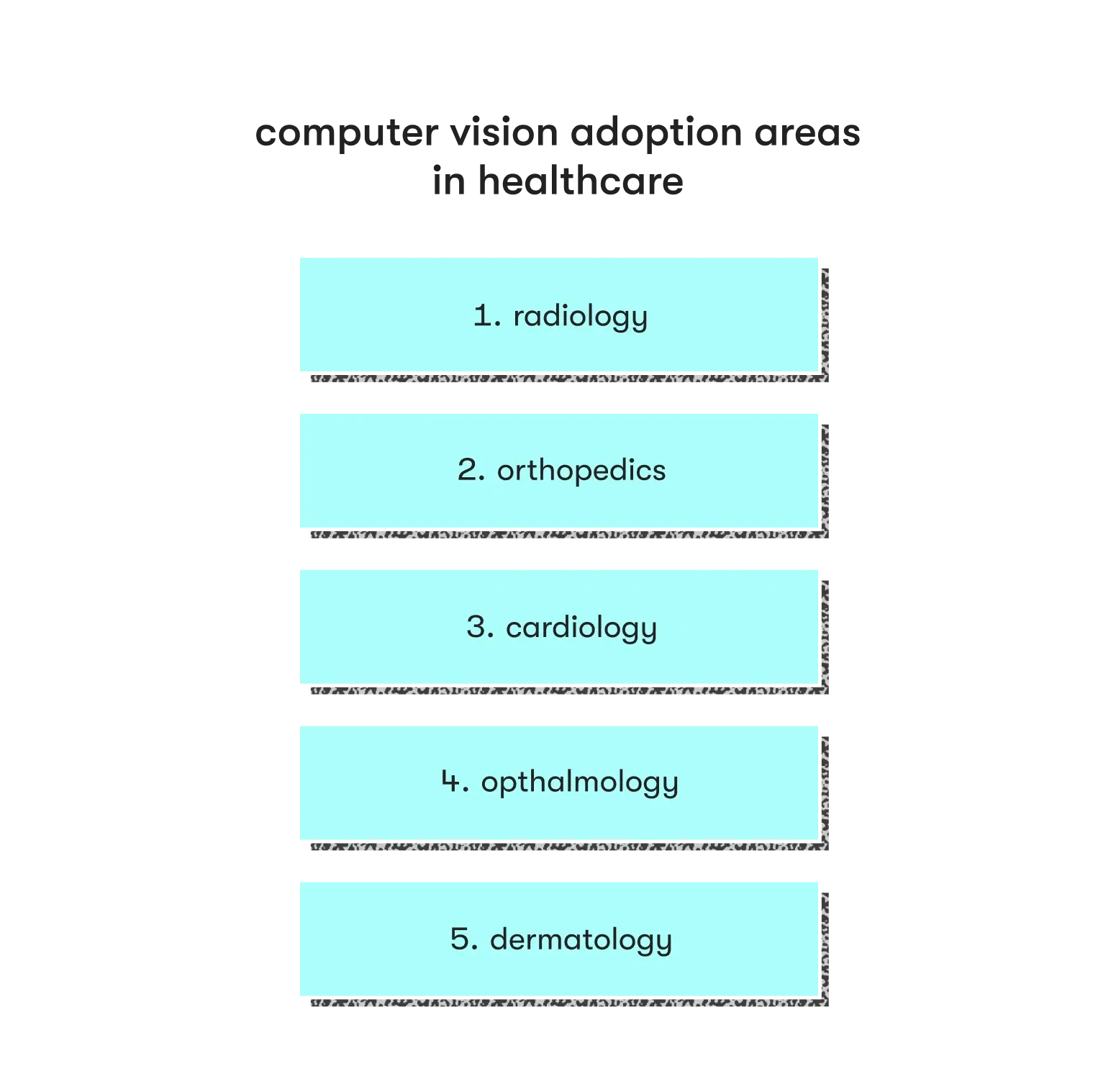
Radiology
Radiology was one of the very first medical disciplines to adopt CV-powered medical applications. Since radiologists heavily rely on DICOM medical imaging data coming from various sources, the availability of precise object recognition algorithms and computer vision for medical image analysis became a truly invaluable practice for a number of purposes:
- Computer-aided inspection of X-ray, CT, and MRI scans of internal organs
- Detection of microscopic bone fractures
- Long-term monitoring of treatment results and change detection
- Early-stage detection of new tissue formations, including tumors
So far, full diagnostic automation is not possible and real doctors will always have the final say, but the combined effort of an experienced clinician with a trained human eye and a powerful machine learning algorithm can yield great results.
Orthopedics
Orthopedics is another area that puts computer vision in medicine to good use, covering the entire range of preoperative, intraoperative, and postoperative activities. The scope of possible applications for AI/DL/CV tools in this field spans a broad variety of operations:
- Surgery planning and intraoperative navigation
- Integration with robotic surgery planning software
- MRI-based arthroplasty planning
- CT, X-ray, MRI segmentation, 2D/3D landmark detection
Cardiology
In cardiology, computer vision aids surgeons and other medical staff in various aspects of their work:
- Helps detect heart development anomalies and monitor the progression of congenital heart diseases
- Helps visualize arteries and blood flow during surgeries
- Helps perform quantitative analysis of cardiac variables
- Approximates blood loss during surgeries
Ophthalmology
Computer vision just could not stay away from vision itself. While consumer grade apps like Cradle help detect early stages of eye diseases in children, similar, yet more sophisticated algorithms are used in clinics for a number of purposes:
- Retina scans and subsequent analysis
- Early-stage detection of eye development anomalies
- Pre-operative planning
- Eye-tracking for eyesight correction
Dermatology
Computer vision was a natural choice for dermatologists, especially those specializing in skin cancer diagnostics and treatment. Doctors can now come up with an accurate medical diagnosis based on a series of photo/video observations of a particular skin formation that were analyzed by a deep learning algorithm trained on thousands of cases of confirmed cancers and benign formations.
Here is how computer vision is used in dermatology:
- High-resolution scanning of skin formations and monitoring of any growth
- Detection of early signs of skin diseases
- Development of personalized skincare treatment plans based on skin type, sensitivity, and reactions to different drugs.
EPAM Startups & SMBs experience in using computer vision in healthcare applications
EPAM Startups & SMBs offers a wealth of experience in the field of artificial intelligence, deep machine learning, computer vision, and healthcare website development services.
We combine this niche experience with our vast healthcare software engineering expertise and an ever-growing global pool of IT specialists that can be quickly assigned to projects of any complexity.
Mindray: Revolutionary Patient Monitor EPAM collaborated with Mindray to build an advanced patient monitoring system. Designed to provide critical information and easy access to healthcare professionals in various environments, this series of monitors features breakthrough products offering a user experience unrivaled by other products on the market. | Life-Saving Software Solutions for CardioReady CardioReady uses a patented process to offer turnkey Certified Cardiac Emergency Response Programs to organizations across the US. EPAM worked with CardioReady to create two applications: Maintenance Minder and Inspector Mobile, as well as a global dashboard to monitor, inspect and assess AED programs. | Poison Emergency App and Digital Triage Tool Unfors RaySafe is the leading provider of solutions to measure, monitor and manage X-ray radiation with a number of international offices. EPAM developed RaySafe S1, the first-ever cloud-based solution for dose management with a broad variety of advanced functionality. |
NEED A TEAM OF COMPUTER VISION TOP GUNS TO IMPLEMENT AN INNOVATIVE HEALTHCARE PROJECT?
You can’t go wrong with EPAM Startups & SMBs. We are a versatile software engineering platform with hundreds of professional business analysts, systems architects, senior developers, data architects and data scientists ready to help you build a health startup or revamp an existing product. Don’t miss your chance to hire some of the best AI developers in the industry!
Conclusion
When it comes to computer vision, healthcare is more than likely to be one of the locomotives of progress in this field. With thousands and thousands of successful implementations of machine learning and computer vision systems in various healthcare practices, this experiment has proved to be extremely successful and truly life-saving, both for patients and overburdened medics.
Computer vision is used across the entire range of patient/physician/hospital interactions, from initial screening to ongoing treatment, planned and urgent surgeries, as well as fast and effective post-recovery patient care.
Computer vision is now a very effective tool capable of dramatically improving a variety of processes and medical operations. The quality and success of particular implementations depends primarily on the experience and technical abilities of the chosen vendor and their custom medical solutions developers, the choice of the most appropriate hardware, ML/DL algorithms, and availability of high-quality, curated datasets.
FAQ
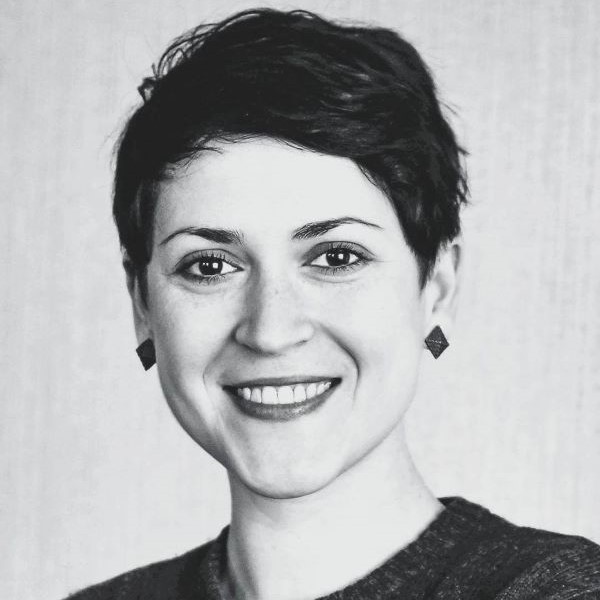
Expert digital communicator and editor providing insights and research-based guides for technology buyers globally.
Expert digital communicator and editor providing insights and research-based guides for technology buyers globally.
Explore our Editorial Policy to learn more about our standards for content creation.
read more