- Big data is growing exponentially and is expected to hit a whopping 149 zettabytes by 2024
- 4 out of 5 consumers are looking for deep personalization of services provided by fintech companies
- Big data analytics in fintech and innovative methods of credit risk assessment open the door to a vast segment of the underbanked, creating a source of new revenue streams
- The financial industry in general still has a long way to go before becoming truly customer-centric, so there exists a world of opportunities here
- Big data and AI are steadily becoming the staples of the modern fintech industry
Take a deep dive into the interplay between fintech and big data analytics to learn how the latter affects the digital transformation in finance.
The role of big data in fintech
Gone are the days when an account statement from a bank could tell the story of your financial life. The portrait of a modern consumer is infinitely more detailed and multi-faceted, spanning not just transactions but a multitude of other factors, such as an array of personal details, online searches, shopping habits, media consumption patterns, social network activities, travel frequency and geography, general areas of interest, and much more.
In order to take advantage of this information, financial companies combine what they already know about their customers with data collected from multiple sources to better understand the characteristics of particular individuals and customer cohorts. With this knowledge, they can not only customize and personalize their offers but also assess risks with higher accuracy and keep their P/L statements in check.
So where does big data come from and what is it in the first place? In short, big data is an overarching term that describes extra-large and constantly growing arrays of diverse (both in terms of nature and structure) data gathered from a variety of channels that are hard to manage in a more raw, unprocessed form. One of the fundamental characteristics of big data is the steady growth of its volume and complexity.
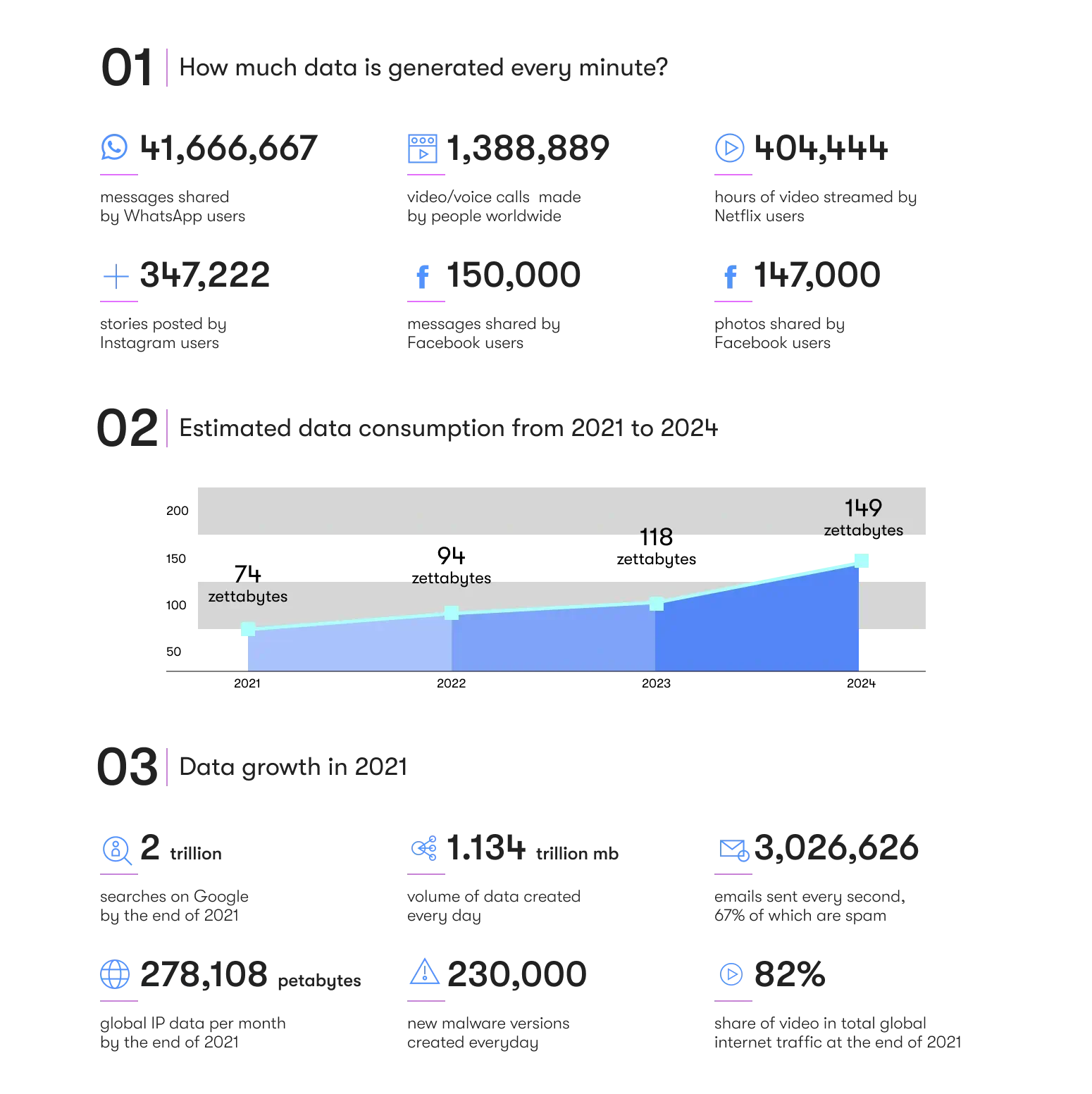
As seen in the illustration above, with around 1.1 trillion megabytes of data generated every day by nearly 4.7 billion Internet users, big data is something that the financial sector simply cannot ignore. And if it’s that high up on the agenda, fintech companies need to move fast to address the three V’s of big data: Volume, Velocity, Versatility. In plain English, they need to build data analytics platforms and adopt new techniques to process more data or different types in the shortest period of time — ideally, in real time.
Interested in learning more about solutions featuring real time data analytics?
Contact us for more information on projects we delivered for Big Data fintech startups and large companies.
Why the financial services industry is having a hard time with modern customers
The importance of big data in the fintech industry becomes even more apparent if we take a deeper look at user demographics. According to recent studies, Millennials are now the driving force behind the rapid development of fintech services. They are skeptical about the ways banks communicate with them and look for more:
- 80% of customers consider a personalized experience provided by financial companies to be just as important as their products and services;
- 66% of customers expect businesses to predict their needs and expectations;
- as many as 73% would choose a neobank or a financial service over a traditional bank for payments, savings, or trading.
In addition, this lack of affinity for conventional banking often results in poor credit scores and histories of a large percentage of Millennials, seriously limiting their access to loans and popular financial tools. As many as 50% of Millennials may be affected by this problem, analysts say. Traditional financial services are still falling short of understanding their target audiences.
All of the above calls for a serious revision of old strategies and a more extensive use of big data analytics in fintech.
How big data helps fintech companies and startups to better serve and protect their customers
There are several distinct areas where financial technologies and big data form a perfect symbiosis that leads to significantly better outcomes for service providers and consumers alike.
Big data processing
- Real-time data processing facilitates the task of tracking users’ activities in web and mobile applications for subsequent analysis.
- Data mining helps take a deeper dive into large volumes of unstructured data stored in data lakes, drawing actionable insights from them.
- Data visualization on customizable dashboards provides a way to get a clear view of the most important aspects of key business processes.
- Stream computing systems merge data streams from applications and Internet of Things networks, analyzing them on the go and returning clean, usable data sets.
Data analytics
- Advanced risk analysis algorithms rely on artificial intelligence and machine learning methods to achieve better accuracy.
- Data-driven decision making is based on big data analysis and AI/ML algorithms that suggest the optimal course of action in complex situations.
- Business process automation coupled with intelligent decision-making reduces service delays and improves the customer experience.
- Continuous social analytics help monitor the brand image in social networks and detect issues at an early stage, thus enabling marketing teams to maintain a consistently high CSAT (customer satisfaction) score.
Data security
- Big data helps build very detailed customer profiles that are used for reliable protection of users’ identity and personal data, which is especially important for financial institutions.
- AI and big data are widely used for detecting suspicious activities and preventing various types of fraud and elaborate hacking attempts.
In principle, these and other related practices and approaches form the data management framework of any modern digital bank or financial technology company. Big data collection and 360-degree data analysis are no longer viewed as a fad that reflects the latest fashion in computer science. On the contrary, they are essential components of any institution working with money and striving to be effective in a very competitive landscape.
Wondering how the AI + big data combo could be used to enforce your financial startup idea?
We have the answers to your questions and will gladly share our thoughts with you.
Big data use cases in fintech: scenarios for financial startups and companies
Big data in fintech companies is truly ubiquitous and actively used in different contexts for a broad range of purposes. Let’s take a look at a few examples of where combining the use of big data and associated technologies makes the most practical sense.
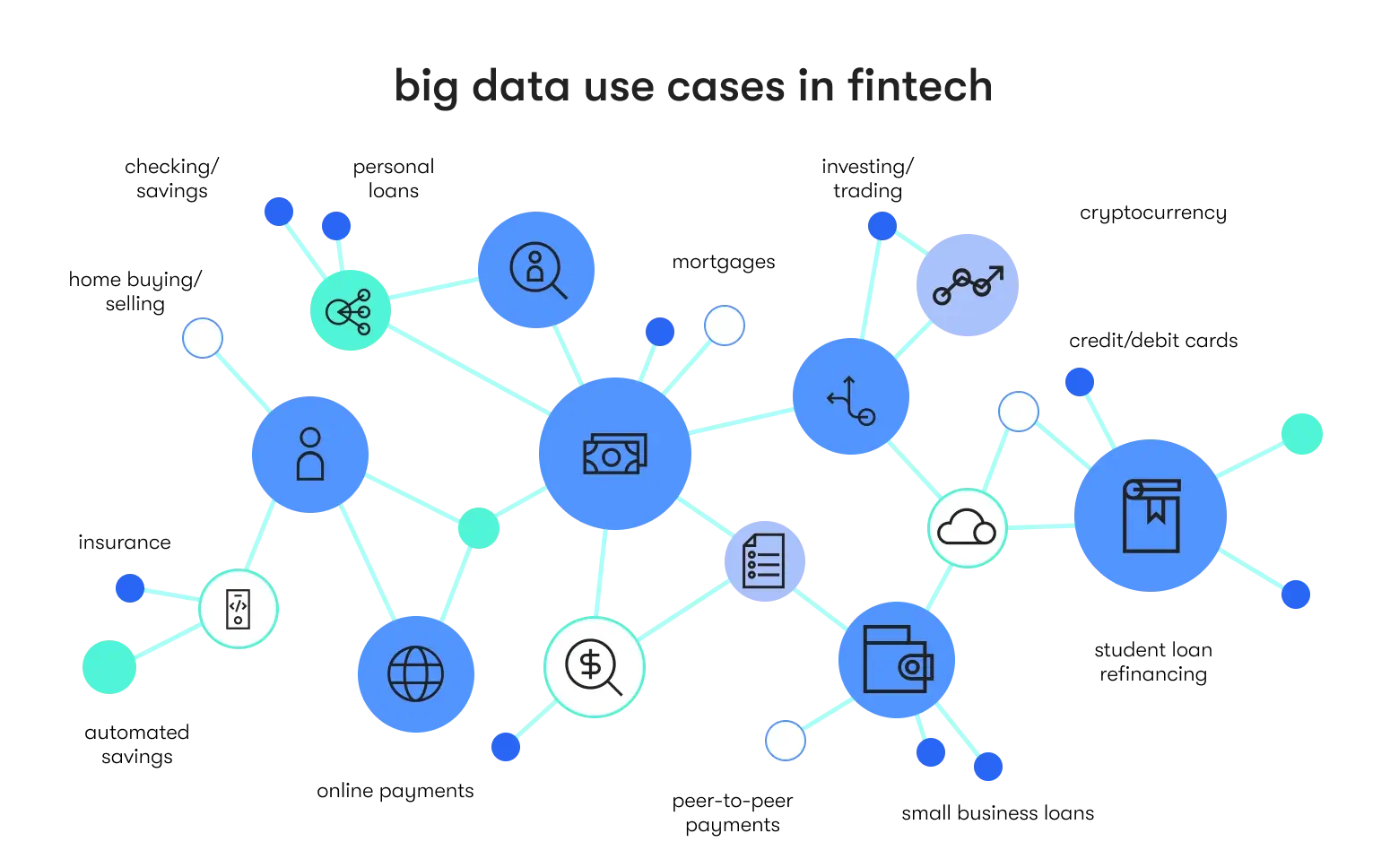
Big data in online payments
Big data and ML-based analytics have been used in the industry for security and fraud detection purposes for quite a while. However, the more recent trend in online payments is the merger of payment processing with POS (Point Of Sale) lending mechanisms that enable users to get loans right at checkout. These systems combine ML algorithms and big data to instantly assess risk levels and the amount of credit available to the user, resulting in fewer cases of abandoned carts and higher conversion rates.
To take one example, the US-based SoFi.com uses all of the above-mentioned technologies to target young professionals and help them pay, save, invest, and borrow money in the most effective and secure way. The market is ready for next-gen financial platforms, and now is a great time for startups with innovative business models and a unique view on how consumers should be handling their finances online.
Big data in insurtech
A lot of insurance companies still don’t use available data insights to create their products and services. On many occasions, they rely on demographic and statistical data that is outdated and no longer relevant. Because of this, they have a hard time setting optimal prices on their policies and often miss out on substantial financial opportunities that simply get overlooked. Modern insurance companies take full advantage of big data and actively use machine learning to create highly customized, low-risk insurance offers that address the specific needs of particular categories of users.
One great example of a service that is changing the car insurance scene today comes from the Swedish company Greater Than. Using massive amounts of literally on-the-ground information and road accident statistics, their business skillfully applies machine learning to aid insurance companies in estimating risks and adjusting pricing levels.
Big data in lending
Big data and AI models are widely used in microfinancing and other types of lending businesses to reduce the cost of credit underwriting and make loans available to a wider audience that often has a challenged credit history. This promotes financial inclusion on the one hand and results in higher revenues for the insurer on the other. In addition, the wider accessibility of instant loans helps boost the economy in general and the business efficiency of small and medium enterprises in particular.
The number of POS financing companies and their revenues are growing at a steady rate. Market leaders like Klarna and Affirm are now facing serious competition from a new wave of POS lending startups, so now could be a great time to join the race with a focus on developing countries where the presence of traditional banking services is limited.
Big data and financial technology in real estate
The priorities of real estate fintech companies are two-fold. First of foremost, they want to sell more with a higher margin, and this goal can be achieved through dynamic pricing, continuous market monitoring, provision of comprehensive information about properties in corresponding listings, and minimizing the risk of their clients defaulting on payments. To this end, they focus on capturing data from various sources and applying data analytics to make the right offers to the right people at the right time.
The other aspect of their business is retention. Leveraging the latest advancements in mobile technology, they can deploy low-power, low-maintenance IoT devices across rented properties as well as offer 24/7 infrastructure monitoring services. With detailed information constantly transmitted to the back office, they can optimize rental and maintenance offers dynamically and keep their customers happy for years.
In a comprehensive study by Deloitte Center for Financial Services, the authors conclude that “Companies can combine, analyze, and present insights from the large sets of data in a manner that tenants or other stakeholders can purchase and augment their actions and behavior.” This creates a fertile soil for startups employing this financial model to create IoT-based facility management solutions with multiple monetization models.
Big data in trading and investing
As trading is becoming increasingly complex with cryptocurrencies and crypto-derivatives being thrown into the mix, staying efficient on a day-to-day basis has become a real challenge. This industry has long relied on data science, and this synergy will continue to evolve as the amount of data processed by trading platforms grows and the reaction time becomes a vital factor, especially in large-volume trading in highly-volatile markets. The adoption of real-time big data analytics in finance and the application of self-learning algorithms now define the varied landscape of online trading platforms.
For example, Goldman Sachs uses ML algorithms and NLP (Natural Language Processing) components to not just perform quantitative analysis of investment candidates, but to also assess the media context and tonality of the public discourse in relation to them, thus achieving a higher accuracy and efficacy of investments. The same mechanisms can be applied by smaller businesses for brand monitoring and adjustment of trading strategies.
How can big data in fintech influence the customer experience?
Now that we’ve covered the major areas in fintech and the big data analytics used in them, let’s focus on the specific ways in which these technologies and approaches can become a game changer for fintech institutions and their customers.
Customer segmentation
Customer segmentation is a part of Marketing 101 — and a very important one at that. In simple terms, it is the process of singling out different groups of customers based on either their actions/behaviors or specific characteristics, such as location, age, gender, marital status, income, education, job type and industry, just to name a few.
Data scientists apply a variety of methods, such as decision trees and clustering, to break down the entire pool of customers into groups with distinct properties. With this data, they can approximate the CLV (Customer Lifetime Value) of every customer or customer group, their appetite for investment risks, duration of mortgage repayment, and propose the best services for each category.
However, businesses are not the only beneficiaries of data analytics. Thanks to this data-driven approach, customers enjoy the improved relevance of offers, special loan repayment terms and other bonuses, and reciprocate with higher loyalty and lower churn.
Service personalization
Service personalization is the cornerstone of today’s fintech and something that the overwhelming majority of customers want to experience. For businesses in this field, the process consists of several steps:
- Initial data collection, customer segmentation/profiling
- Making the data available throughout the company in a unified format
- Creation of personalized products and services in several areas:
- Long-term personalization: forecasting of customers’ needs and generation of highly relevant, personalized content delivered across a variety of channels;
- Real-time personalization: for example, shopping or trading recommendations through mobile apps or browser extensions;
- Personalization of communications: for example, through AI-driven bots fulfilling 24/7 support functions and having access to the history of prior communications and the customer’s profile.
Customer support
Whether happy or unhappy, customers do not always share their feelings directly with their service providers. With big data and AI, companies can now crawl social networks and hear the voices of their customers more clearly. This results in faster responses to reported issues and the subsequent creation of a positive image of the company thanks to a public demonstration of high customer care standards.
Compliance
The financial industry is heavily regulated at many levels and is subject to frequent audits and certifications that result in a high managerial overhead and resource drains. With big data and AI used strategically, fintech companies have a bird’s-eye view of relevant parameters and can be better prepared when the time comes to reconfirm their adherence to norms and regulations. Their customers, in turn, benefit from having services provided by a trusted financial partner.
Ready to share your ideas and see if EPAM Startups & SMBs is up to the challenge?
Our team of technology and domain experts is always a phone call away.
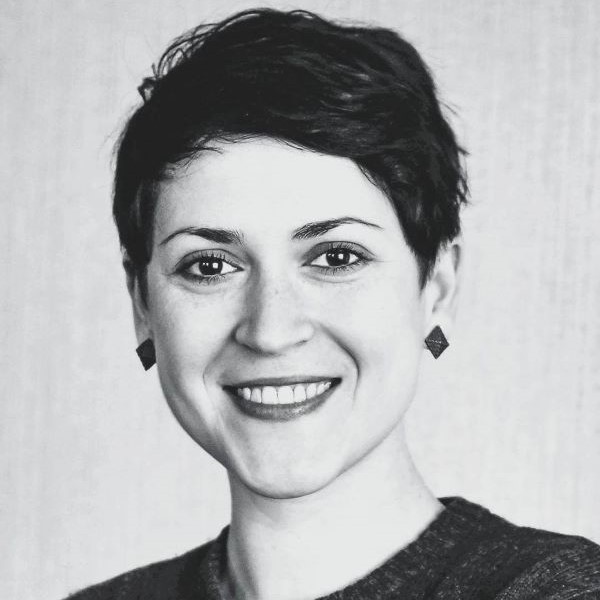
Expert digital communicator and editor providing insights and research-based guides for technology buyers globally.
Expert digital communicator and editor providing insights and research-based guides for technology buyers globally.
Explore our Editorial Policy to learn more about our standards for content creation.
read more