In recent years, the banking industry has witnessed a significant transformation with the advent of artificial intelligence in fintech. This article provides a comprehensive overview of the state of AI banking, highlighting the types of AI use cases, challenges, current use cases, and emerging trends.
The current state of AI in the banking sector
The banking industry has traditionally been the driving force behind implementing innovative solutions on a large scale — for instance, the introduction of ATMs in the 1960s or the adoption of mobile banking apps in the previous decade. As of today, disruptive artificial intelligence (AI) technologies enable banks to pursue four main goals:
- Personalize customer experiences
- Offer unique omnichannel interactions
- Accelerate innovation cycles
- Increase profitability
As of 2024, AI has transformed various aspects of banking, from customer service and risk management to fraud detection and personalized financial advice. A Business Insider report indicates that 75% of banks with over $100 billion in assets implemented AI-centered strategies and tools in 2022.
Conversational and generative AI in banking
Conversational AI (CAI) in banking encompasses the implementation of artificial intelligence technologies such as natural language processing (NLP), natural language understanding (NLU), and machine learning to enable human-like interactions between banks and their customers. Conversational AI involves using chatbots, virtual assistants, and voice-enabled systems that can understand and respond to customer queries, provide information, and assist with various banking services.
Conversational AI solutions in banking fall under three main types depending on their purpose.
- Virtual assistants: These are personal assistants (like Amazon Alexa, Google Assistant, and Siri) designed to interact with customers and assist them in various banking-related tasks, such as personalized customer support, account management, product recommendations, and appointment scheduling.
- Virtual agents: Although somewhat similar to AI-powered assistants, virtual agents act as bank representatives to fulfill various business objectives. A prominent example of a virtual agent is Capital One's Eno, engaging with customers to provide banking-related services and support.
- Natural language processing (NLP) systems: NLP systems enable banks to interpret and analyze unstructured customer text data, such as emails, chat logs, or social media messages. NLP systems help extract the main intent from customer communications, allowing banks to identify customer needs and respond effectively.
Generative AI (GAI) in banking refers to the application of generative language tools and models (think ChatGPT or DALL-E) to create new and original data or content within the banking sector. Generative AI models can learn from existing data patterns and generate new outputs that mimic the characteristics of the training data.
Generative AI in banking falls into different types based on its specific applications and techniques.
- Generative adversarial networks (GANs): GANs are a popular type of generative AI model that consists of two components: a generator and a discriminator. GANs are trained in a competitive manner, meaning that the generator creates synthetic data samples and the discriminator distinguishes between real and generated data. Banks use GANs for tasks such as generating synthetic transaction data for fraud detection or creating synthetic market data for risk assessment.
- Language generation models: Language generation models, such as OpenAI's GPT, are widely used in banking for tasks related to content generation and natural language processing. These models are trained on large text corpora and can generate coherent and contextually relevant textual content. Banks use such models to offer personalized financial advice, automate reports, and improve customer communication.
- Market simulators: Market simulators leverage generative AI techniques to create synthetic market data that mimics real-world market conditions. These simulators generate market scenarios and data to assess portfolio performance, optimize investment strategies, and perform stress testing, enabling banks to evaluate the potential impact of different market conditions on their portfolios.
- Synthetic data generation: Generative AI for banking is used to create synthetic data that closely resembles real customer or transaction data while preserving privacy. This synthetic data allows banks to test and train AI models, conduct data analysis, or share data with their external partners without compromising sensitive customer information.
Key takeaways:
Although both types can be applied independently, combining generative AI with conversational AI provides the maximum value. Conversational AI ensures seamless and guided conversations, providing essential boundaries for generative AI. Moreover, CAI enriches interactions with customized company-specific responses, offering valuable information such as operating hours, office locations, and protocols.
In turn, generative AI brings creativity, fluidity, and adaptability to the conversation. This dynamic interplay between these two AI approaches results in a transformative experience, empowering banks to achieve new levels of efficiency and engagement with their audience.
LET’S BUILD YOUR AI-DRIVEN BANKING SOLUTION TOGETHER
Examples of artificial intelligence in banking
As customers increasingly rely on digital channels in their daily routines, they expect from banks the same level of convenience, speed, and personalized service provided by digital-native companies.
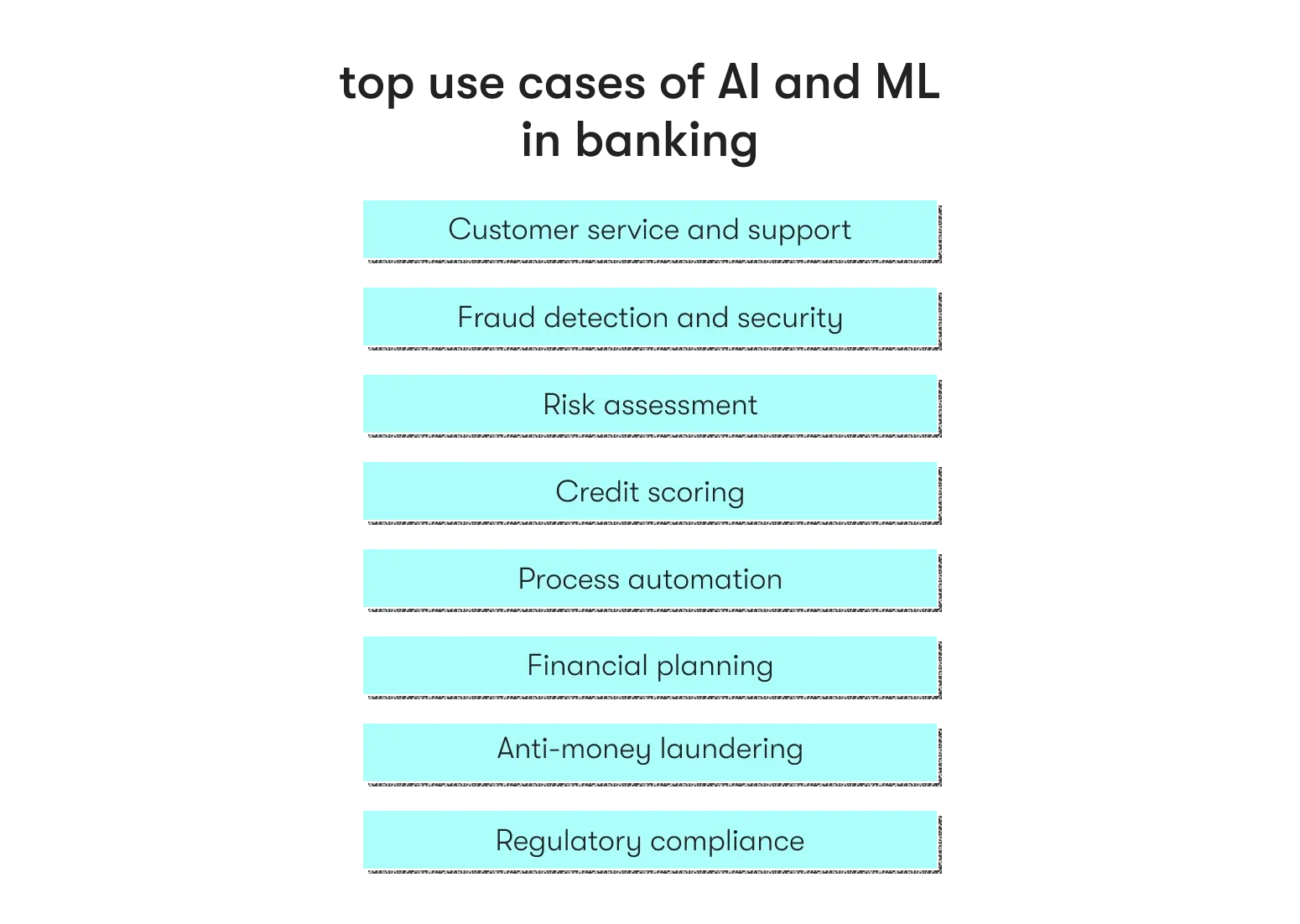
Brick-and-mortar banks should establish a fresh value proposition built on AI and analytics capabilities to remain competitive in this demanding landscape. In this regard, it is crucial to ensure that the customer experience is contextual, personalized, and customized, and this is precisely where artificial intelligence steps in.
The current application of AI in banking encompasses several areas.
- Customer service and support: AI-powered chatbots and virtual assistants provide round-the-clock support, addressing customer queries and offering personalized recommendations.
- Fraud detection and security: AI algorithms can analyze vast volumes of data, detecting patterns and anomalies to identify potential fraud and enhance security measures, powered by cloud banking solutions.
- Risk assessment and credit scoring: AI models analyze customer data, credit history, and other variables to accurately evaluate creditworthiness, expediting loan approvals and improving risk assessment.
- Process automation: AI technologies automate manual and repetitive tasks, such as data entry, document verification, and compliance checks, reducing errors and increasing operational efficiency.
- Financial planning: AI algorithms analyze customer financial data and behavior to provide personalized investment, savings, and budgeting recommendations. By considering individual goals, risk tolerance, and market conditions, AI-powered systems can offer tailored financial advice, empowering customers to make data-driven decisions.
- Anti-money laundering (AML) and regulatory compliance: AI systems can analyze large volumes of transactions and data to detect patterns indicative of money laundering or regulatory violations. By automating AML processes, banks can enhance compliance, reduce false positives, and mitigate financial risks.
AI and banking: real-life cases
As mentioned earlier, Capital One stands out as a leading example of AI integration in personal banking with its intelligent virtual assistant Eno. Eno is an innovative tool that provides users with a range of helpful features and services to manage their finances and credit card accounts more efficiently.
Eno leverages AI to analyze users' spending patterns and provide personalized insights and recommendations to help manage their finances more effectively. For instance, Eno is instrumental in enhancing security measures for credit card transactions. The virtual assistant can proactively monitor and identify suspicious charges, notifying users of potential fraudulent activities.
JPMorgan Chase has likewise made significant strides in leveraging AI and deep learning techniques to enhance cybersecurity. Their researchers have developed an impressive early warning system that swiftly detects malware, trojans, and phishing campaigns. By providing timely alerts to the cybersecurity team, the bank can thwart attacks before they cause significant damage.
Their other product, OmniAI, is a platform that accelerates the extraction of valuable insights from vast amounts of data, streamlining the process for the firm's data scientists.
The platform's remarkable efficiency in reducing "time to insight" translates into quicker and more accurate decision-making, empowering businesses to respond promptly to market dynamics and evolving customer needs. With OmniAI, teams can delve deeper into data, conduct comprehensive analyses, and uncover hidden patterns that were previously elusive.
A standout advantage of OmniAI is its cost-effectiveness, significantly lowering operational expenses while boosting analytical capabilities. Businesses can optimize their resources by efficiently handling large volumes of data, focusing on better serving their clients and customers.
Speaking of the robo-advisor sector, Wealthfront is regarded as a prominent market leader, boasting an impressive user base of 440,000 individuals and managing a substantial $25 billion in assets. The platform offers diverse investment options, including cryptocurrencies, catering to investors with varied preferences.
One of the standout features of Wealthfront is its commitment to maintaining meager fees. Most accounts incur a minimal charge of only 0.25%, and users benefit from fee-free transactions. This combination of accessibility, a wide array of investment choices, and general cost-effectiveness have contributed to its significant success in the market.
Similar to Wealthfront:
Betterment: Founded in 2008, Betterment is a comprehensive financial management platform encompassing automated investments, financial planning, and cash accounts. As one of the pioneering companies in the robo-advisor space, Betterment has been at the forefront of revolutionizing investment services.
M1: Established in 2015, M1 has earned the moniker of "The Finance Super App." This dynamic platform, similar to Wealthfront, provides various financial services, including investment opportunities, savings options, banking services, and loan offerings. This multifaceted approach has made the platform a prominent player in the financial technology arena.
Beyond commercial banks, investment banks like Goldman Sachs and Merrill Lynch have also embraced AI-based tools to streamline their banking operations and drive innovation. Among these tools, Alphasense, an AI-driven search engine employing natural language processing, has proved invaluable in discovering market trends and analyzing keyword searches. The platform is trusted by over 4,000 enterprise-level clients, including a majority of the S&P 500.
The benefits of AI in banking
As seen in the examples above, the greatest financial effects from AI technologies are tangible in customer service, credit scoring, and detection of fraudulent transactions.
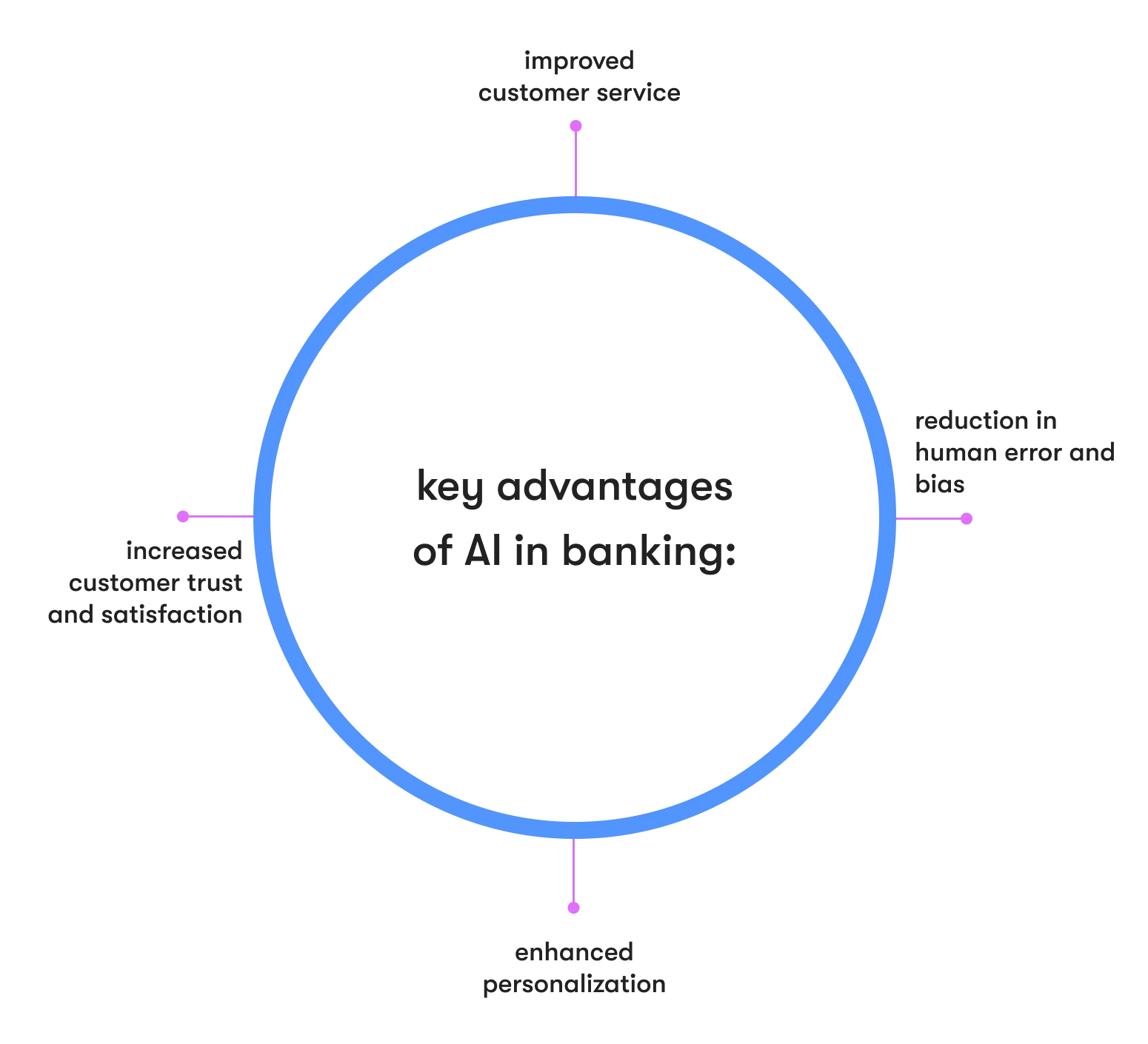
- Improved service response: AI enables faster and more efficient customer service, with automated systems capable of handling inquiries, providing instant responses, and offering round-the-clock support.
- Reduction of human error and bias: AI algorithms minimize the risk of human mistakes and bias that may occur in manual processes, leading to more accurate and reliable results.
- Enhanced personalization: AI empowers banks to deliver personalized experiences by analyzing customer data, preferences, and behaviors. This approach results in tailored product recommendations, streamlined onboarding, customized offers, and targeted marketing campaigns.
- Increased customer trust and satisfaction: With AI's ability to provide quick, accurate, and personalized services, customers experience enhanced satisfaction and confidence, leading to stronger customer relationships with their banks.
LET’S BUILD YOUR AI-DRIVEN BANKING SOLUTION TOGETHER
Challenges in implementing AI in banking
Big data in banking plays a pivotal role in banking and finance, and AI has emerged as a powerful tool for analyzing extensive datasets, yielding valuable insights that empower financial institutions to make informed decisions.
At the same time, implementing AI comes with its own data-related challenges, including data privacy and banking cybersecurity, regulatory compliance requirements, and the need to upskill the workforce.
The current challenges of implementing artificial intelligence for banks include the following:
Data quality and availability
AI relies heavily on data, and the quality and availability of relevant data is often regarded as the biggest challenge. Inaccurate or incomplete data often leads to biased or unreliable predictions, potentially resulting in flawed decision-making processes. Banks must invest in data cleansing, validation, and enrichment processes to ensure that the data used to train AI models is accurate, consistent, and error-free.
Data silos
In addition, banks often operate with multiple systems and data sources as part of their bank IT services, resulting in data silos. This fragmented data landscape hinders the seamless integration and availability of relevant data for AI training. To overcome this challenge, banks should focus on unifying their data sources and creating a centralized data repository that enables a comprehensive view of customer information, transactional data, and other relevant financial information.
Data collecting and sharing
Banks need to collect and use data in compliance with all legal norms. This is a challenging task since the banking industry is quite heavily regulated. Therefore, banks must prioritize implementing robust security measures in chat interfaces, protecting sensitive data from unauthorized access and disclosure.
Regulatory compliance
Banks operate in a highly regulated environment, and implementing AI requires ensuring compliance with various regulations, such as data privacy (e.g. GDPR) and financial regulations (e.g. KYC and AML).
Ensuring that AI systems meet regulatory requirements while maintaining transparency and explainability is a complex issue, and many banks have a hard time dealing with regulators.
The majority of scoring models that most banks use are based on neural networks and decision tree compositions. The problem with this is that the detailed description of algorithms is so complicated that they are often called "black boxes." This lack of transparency can be problematic from a regulatory perspective, as regulators need to understand precisely how certain decisions are made.
Talent gap
Building high-quality models requires deep expertise in understanding algorithms, tools, and banking processes. In this regard, the lack of qualified specialists in areas such as AI, machine learning, and data analysis poses a serious challenge for banks and fintech companies.
Customer adoption
Banks must proactively raise awareness among customers regarding their digital products and their benefits while ensuring comfort in actually using them. Achieving this objective will necessitate additional considerations in product UX design and investments in educational efforts to create easily accessible and user-friendly interfaces.
Overall, addressing these challenges requires a comprehensive strategy that includes data management, regulatory compliance frameworks, ethical guidelines, effective change management, customer education, and robust risk management practices.
How to evaluate AI project success in banking
Evaluating the success of an AI project in banking requires considering various factors that align with the project's objectives and desired outcomes.
Here are some key aspects to evaluate AI project success in banking.
- Business goals: Assess whether the AI project has achieved its intended business goals. This could include metrics such as cost savings, revenue generation, process efficiency improvements, customer satisfaction enhancement, and risk reduction. Measure the project's impact on the overall business objectives and determine if it aligns with expectations.
- Performance metrics: Define specific performance metrics to measure the AI project's success. These metrics could be related to accuracy, response time, throughput, error reduction, or any other relevant factors that demonstrate the effectiveness of the AI solution. Compare the project's performance against predetermined benchmarks or industry standards.
- User adoption and satisfaction: Evaluate user adoption and satisfaction levels. Collect feedback from users such as employees or customers and evaluate their experience with the AI system. Assess factors like usability, ease of interaction, perceived value, and overall satisfaction.
- ROI and cost-benefit analysis: Conduct a comprehensive analysis of the project's return on investment (ROI) and cost-benefit ratio. Assess the financial impact of the AI project, considering factors like initial investment, ongoing maintenance costs, and the value generated or saved as a result. Compare the benefits achieved to the project's costs to determine its financial success.
- Risk mitigation and compliance: Evaluate how the AI project contributes to risk mitigation and compliance objectives. Assess whether the system helps detect and prevent fraudulent activities, enhances regulatory compliance, and reduces operational risks.
- Scalability and future potential: Consider the scalability and future potential of the AI project. Assess whether the solution can handle increasing volumes of data, adapt to changing business needs, and integrate with other systems. Evaluate the potential for expansion into new use cases or areas within the banking organization.
- Continuous improvement: Determine if the AI project has established a process for continuous improvement. Make sure the system is regularly updated, refined, and optimized based on feedback, new data, and emerging technologies. The ability to evolve and improve over time is essential for long-term success.
It's important to establish clear evaluation criteria and metrics before initiating the AI project to ensure effective measurement of its success. Regularly review and assess these factors throughout the project's lifecycle to track progress, identify areas for improvement, and ensure alignment with business objectives.
LET'S MAKE A SUCCESSFUL AI BANKING PROJECT TOGETHER
Emerging AI trends in banking
The banking landscape is radically transforming, creating a future where banking becomes seamlessly integrated into everyday life. As a result, banking services will be ubiquitous, effortlessly accessible, and deeply embedded in our daily routines.
Personalized banking experiences will be further enhanced, enabling more accurate financial planning and tailored recommendations. Additionally, the use of AI in combating financial crimes is expected to evolve, ensuring proactive detection and prevention of fraudulent activities.
The traditional banking industry has witnessed significant disruption due to the emergence of fintech startups. These startups are harnessing the power of AI to create powerful and customer-centric products and services. Looking ahead, we can expect a surge in partnerships between financial institutions and fintech ventures, leading to fresh and inventive applications of artificial intelligence in the banking sector. The future holds great promise for exciting advancements in this dynamic landscape.
As technology advances, AI-powered solutions are expected to become more sophisticated and capable of handling complex finance-related tasks. Although current AI capabilities surpass human performance in specific tasks, the pressing challenge lies in advancing narrow AI toward broader general and super AI.
- Narrow AI (aka weak AI) refers to AI systems that are designed and programmed to perform specific tasks or functions within a limited domain.
- General AI (also known as super or strong AI) refers to AI systems with human-like cognitive abilities, enabling them to understand, learn, and apply knowledge across a wide range of tasks, just as a human can. The path to achieving this lies in the ability of AI to master cross-domain tasks, seamlessly transitioning from one domain to another and constructing new neural networks from scratch.
The active use of AI technologies in the coming years may become a decisive argument in the competition for mass segments. Alex Jimenez, EPAM’s Managing Principal of Financial Services Consulting, advocates for a measured approach to AI. “I think we have to be adults when engaging this technology,” she says. “One of the finest ways to engage AI is to think about it as a co-collaborator… rather than think of it as some, you know, alien other, and to sort of bring it into the mix.”
How to become an AI-driven bank
Becoming an AI-driven bank requires careful planning, strategic implementation, and a commitment to harnessing the full potential of artificial intelligence. Here is a 5-step guide to help you transform your bank into an AI-driven institution.
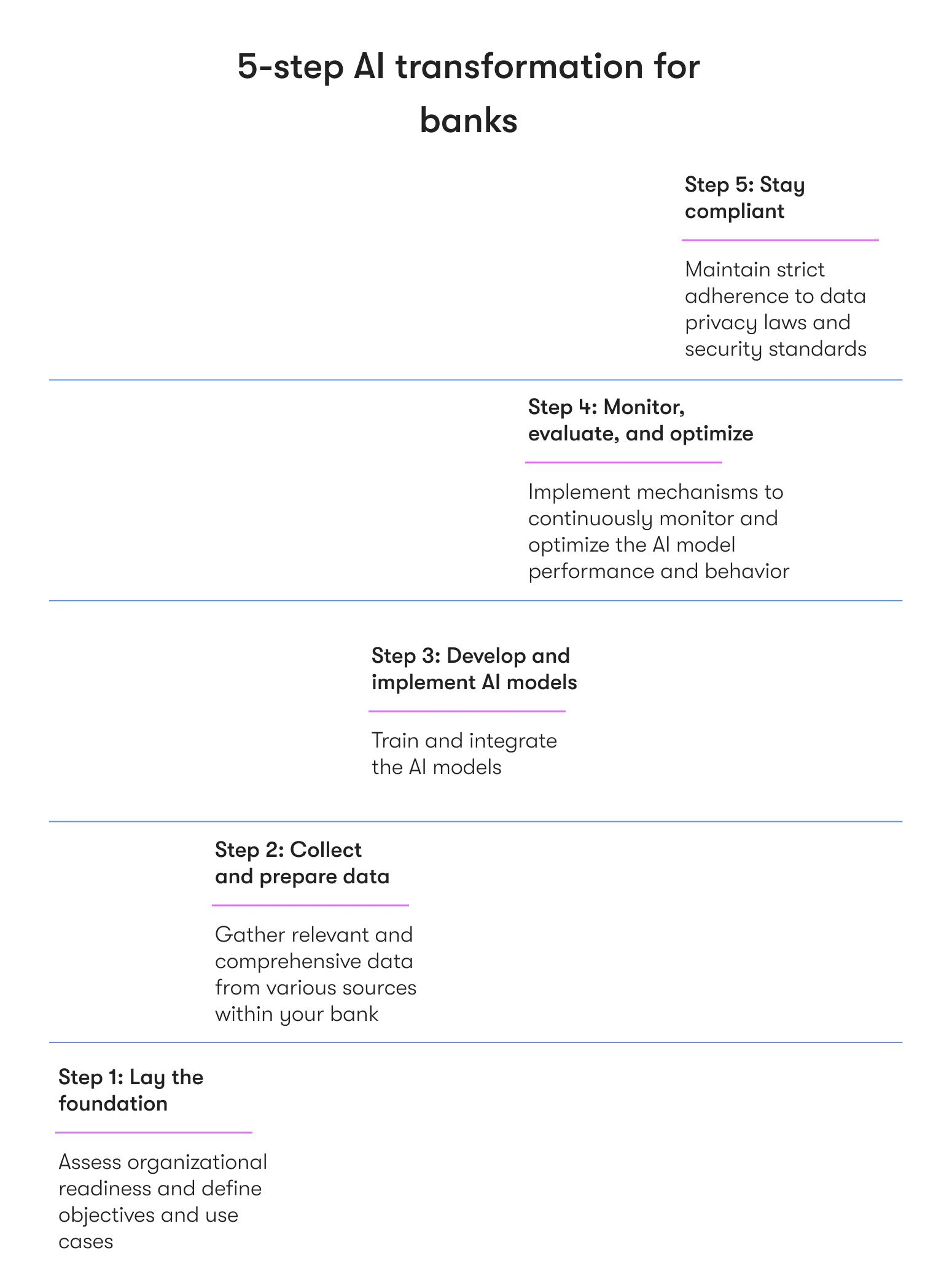
Step 1: Lay the foundation
- Assess organizational readiness: Start by evaluating your bank's current technological capabilities, data infrastructure, and readiness to adopt AI solutions. Identify key stakeholders and create a cross-functional team to lead the AI-driven transformation.
Define objectives and use cases: Clearly outline the goals you want to achieve with AI implementation. Identify specific use cases where AI can have a significant impact, such as fraud detection, customer service, personalized recommendations, or risk management.
Evaluate data management and security: Ensure you have a robust data management strategy in place. Establish data governance policies, quality standards, and compliance measures to protect sensitive customer information and adhere to regulatory requirements.
Step 2: Collect and prepare data
- Data collection: Gather relevant and comprehensive data from various sources within your bank, including transaction data, customer behavior, historical records, and any other data that can help train AI models effectively.
Data cleaning and preprocessing: Prepare the data for AI analysis by cleaning, transforming, and normalizing it. High-quality, well-organized data is crucial for accurate AI model training.
Step 3: Develop and implement AI models
- Hire AI talent: Recruit skilled AI professionals, machine learning engineers, and data scientists to build and implement AI models. Alternatively, consider partnering with AI software engineering vendors like EPAM Startups & SMBs if you lack in-house expertise.
Model selection: Choose appropriate AI technologies and algorithms based on the identified use cases.
Training and testing: Train your AI models using the prepared data and continually fine-tune them to improve accuracy and performance. Thoroughly test the models to ensure they meet the desired objectives and deliver reliable results.
Integration: Integrate the AI models into your bank's existing systems and processes, such as customer service platforms, fraud detection systems, or investment advisory tools.
Step 4: Monitor, evaluate, and optimize
- Continuous monitoring: Implement mechanisms to continuously monitor the AI models' performance and behavior. Regularly check for bias, data drift, and any other potential issues to maintain the models' accuracy and fairness.
Feedback loop: Encourage feedback from customers and internal users to improve the AI systems' usability and address any shortcomings promptly.
Scale and expand: Once you have successfully implemented AI in specific areas, explore opportunities to expand its usage to other departments and processes across the bank. Seek innovative ways to leverage AI and create new value for customers and the organization.
Step 5: Stay compliant
- Data privacy and security: Maintain strict adherence to data privacy laws and security standards to safeguard customer data and maintain trust.
How we can help
With over 15 years of experience working with financial services companies, EPAM Startups & SMBs helps you maximize the benefits of AI adoption in your financial organization. With skilled engineers, consultants, and designers on board, we assist financial organizations in gaining a competitive edge through AI development services while innovating.
We can handle the complete application development lifecycle, catering to various needs and excelling in fintech software development services.
Another of our significant advantages lies in compliance. As a well-established international company, EPAM Startups & SMBs boasts experience across numerous markets and jurisdictions. Consequently, our adept team possesses the knowledge and insight to develop AI-based software that aligns seamlessly with national and international laws and regulations. Your project will be in safe hands with us, ensuring adherence to all necessary legal requirements.
Here’s what we can do for you:
- Our consulting team will help you identify use cases and how conversational/generative AI could impact your bank’s operating costs and revenue.
- Building on the assessment, we help you define your AI strategy and roadmap to identify and prioritize innovations for both short- and long-term impact.
- By working collaboratively and applying a technology-forward approach, we help you envision an optimized future state of your bank.
- Finally, our development team will help you turn that vision into a reality by leveraging our engineering excellence, end-to-end AI product development capabilities, and AI model deployment expertise.
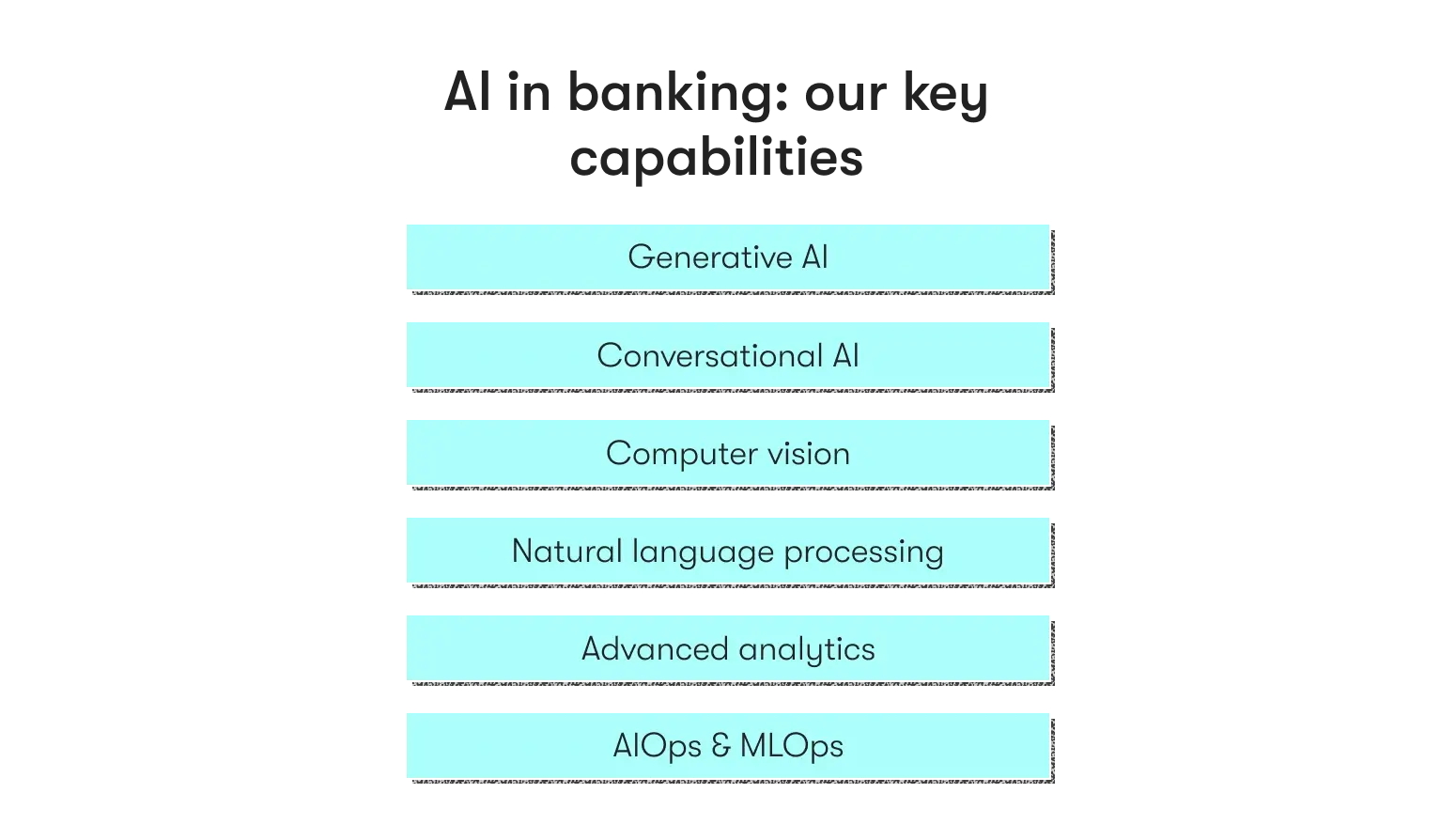
Apart from AI-based services for banking, we also offer end-to-end product engineering and digital transformation services across various financial domains, including asset and wealth management, retail, corporate and investment banking, regulation, risk and compliance, finance, and accounting.
IS YOUR BANK READY FOR AN AI-DRIVEN FUTURE?
Closing words
Banks have recognized the potential of AI in streamlining operations, and the digital transformation in banking is in full swing. At the same time, it would be fair to say that banking AI is going through a period of large-scale piloting and testing.
As mentioned earlier, today we are mainly dealing with narrow AI (ANI) technology — a task-oriented version of AI developed to perform only certain tasks. However, artificial intelligence shows itself as a fairly mature technology in areas like customer scoring and anti-fraud.
While challenges remain, the potential benefits of AI adoption in banking are substantial, and fintech software development is getting more and more attention from the banking industry. As technology evolves, staying informed about current trends, success metrics, and emerging technologies will be crucial for banks that seek to remain competitive in the digital era.
In this regard, decision-makers should treat solutions based on artificial intelligence purely pragmatically and use them to achieve specific goals. Banks can adopt AI into their current systems by following a phased approach, automating routine tasks, and progressively incorporating more intricate processes.
The advent of ethical and responsible AI systems in the banking sector depends on several factors and requires a collaborative effort among banks, regulators, and banking technology providers. This sort of synergy creates a secure and trustworthy AI-driven banking environment that benefits both customers and the industry as a whole.
FAQ
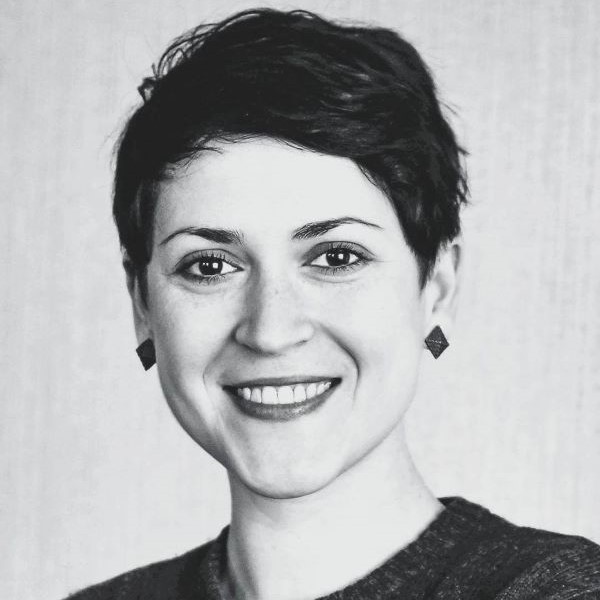
Expert digital communicator and editor providing insights and research-based guides for technology buyers globally.
Expert digital communicator and editor providing insights and research-based guides for technology buyers globally.
Explore our Editorial Policy to learn more about our standards for content creation.
read more